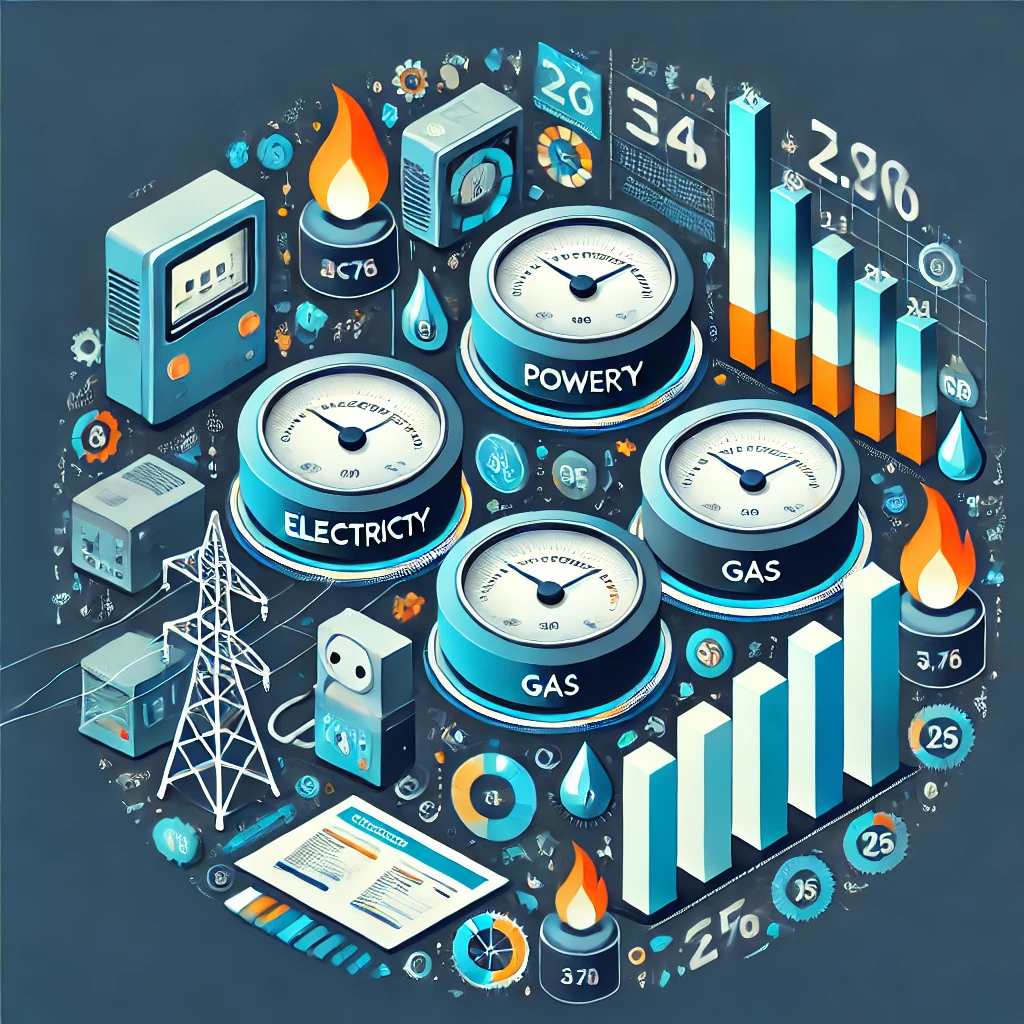
In today’s fast-paced world, where sustainability and cost-efficiency are top priorities, energy data analytics is revolutionizing how businesses and individuals manage their energy consumption. But what exactly is energy data analytics, and why is it so important? More importantly, how can it help you achieve operational efficiency and uncover actionable energy insights? Let’s explore the answers.
What is Energy Data Analytics?
Energy data analytics is the process of collecting, analyzing, and interpreting energy-related data to optimize energy usage, cut costs, and improve overall efficiency.
Think of it as converting raw data—like electricity consumption patterns or equipment performance—into actionable insights that drive smarter energy decisions.
Real-Life Example:
Imagine a manufacturing plant identifying peak energy consumption hours through analytics. By shifting energy-intensive tasks to off-peak times, the plant reduces energy bills significantly while maintaining productivity. This is energy data analytics in action.
Why Energy Data Analytics Matters
1. Drives Operational Efficiency
Energy data analytics identifies inefficiencies such as outdated equipment, wasteful processes, or suboptimal scheduling. Addressing these inefficiencies helps businesses streamline operations, minimize downtime, and enhance productivity.
2. Saves Money
Energy costs are a major expense for businesses. By pinpointing unnecessary consumption, analytics empowers businesses to cut waste, optimize energy contracts, and lower operational costs.
3. Supports Sustainability Goals
With climate change in focus, reducing carbon footprints is essential. Energy data analytics helps organizations track energy usage and emissions, ensuring compliance with sustainability targets and environmental regulations.
4. Enhances Decision-Making
Analytics enables data-driven decisions. Whether it’s about energy upgrades, process optimization, or investment strategies, businesses can rely on insights instead of guesswork.
How to Implement Energy Data Analytics for Operational Efficiency
Here’s a step-by-step guide to get started with energy data analytics:
1. Collect Data
Start by gathering energy-related data from:
- Smart Meters: Provide real-time usage data.
- IoT Devices: Monitor equipment performance.
- Utility Bills: Offer historical energy consumption trends.
The more comprehensive your data, the better your insights will be.
2. Analyze Patterns
Use analytics tools to identify trends in your energy usage.
- Are there specific times when consumption spikes?
- Which machines or processes consume excessive energy?
Answering these questions helps you uncover inefficiencies.
3. Identify Opportunities for Improvement
Once the data is analyzed, pinpoint areas to reduce waste or optimize operations.
- Upgrade to energy-efficient equipment.
- Adjust operational schedules to avoid peak hours.
- Automate systems to eliminate unnecessary consumption.
4. Monitor and Adjust
Energy data analytics is an ongoing process. Continuously track energy usage, measure the effectiveness of implemented changes, and refine strategies to maintain efficiency.
Common Challenges and How to Overcome Them
1. Data Overload
Challenge: Too much data can feel overwhelming.
Solution: Focus on key performance indicators (KPIs) such as peak demand, idle time energy usage, and equipment efficiency. Visualization tools like dashboards can simplify data interpretation.
2. Lack of Expertise
Challenge: Teams may lack the skills to analyze energy data effectively.
Solution: Invest in training programs or partner with energy consultants and analytics providers.
3. High Initial Costs
Challenge: Implementing analytics tools requires upfront investment.
Solution: Leverage government incentives or utility rebates. Remember, long-term savings often outweigh the initial costs.
Real-World Examples of Energy Data Analytics
1. Retail Stores
A national retail chain used analytics to optimize lighting and HVAC systems. By adjusting settings based on occupancy and weather, they reduced energy costs by 20%.
2. Manufacturing Plants
A manufacturing facility discovered that certain machines consumed excessive energy during idle times. By implementing automated shutdowns, they saved thousands of dollars annually.
3. Office Buildings
An office building used energy data analytics to identify inefficiencies in its heating system. Switching to a smart thermostat resulted in a 15% reduction in energy consumption.
The Future of Energy Data Analytics
The future of energy data analytics is being shaped by emerging technologies, offering even greater potential:
1. AI and Machine Learning
AI-powered tools will predict energy usage, detect inefficiencies, and automate energy-saving measures in real-time.
2. IoT Expansion
More advanced IoT devices will provide detailed insights into equipment performance and energy use, enhancing precision in optimization efforts.
3. Self-Optimizing Systems
Imagine energy systems that adjust automatically to changes in demand, weather, or energy prices. These advancements will redefine energy efficiency.
4. Integration with Renewable Energy
Analytics will play a pivotal role in integrating renewable energy sources like solar and wind into grids, ensuring stable supply and demand.
Conclusion
Energy data analytics is no longer a futuristic concept—it’s a necessity for achieving operational efficiency, cutting costs, and driving sustainability. By leveraging energy insights, businesses can make smarter decisions, reduce waste, and stay ahead in a competitive landscape.